Drawing Capital Newsletter
December 30, 2020
In this week’s newsletter, we are exploring the protein folding problem that’s puzzled scientists for over 50 years.
What are Proteins?
Proteins are biomolecules responsible for cell, tissue, and organ functionality. They consist of subunits called amino acids which are the building blocks of life as we know it. When you consume proteins, your body breaks them down into amino acids used to build new proteins.
Amino acids are chained together by peptide bonds to form polypeptides. Polypeptides can either be part of or all of a protein.
Form and Function
Traditionally, in architecture and engineering, the principle of “form follows function” helps us design systems based on what we want the system to do. For example:
Computer keyboards must type English quickly; how do we design that?
Vehicles must get people from A to B safely; how do we design that?
Proteins follow the opposite principle: “function follows form”. A protein’s structure defines the protein’s behavior. If a protein is improperly structured due to a mutation, it can cause disease or other detriments to your body.
Another way of describing a protein’s structure is describing how it folds from a long chain into a 3D origami-like structure.
Folding
Protein folding is the way in which a protein folds from a chain of amino acids into a functional 3D-structure. The chart below illustrates the 4 levels of protein folding.
According to Anfinsen’s Dogma, the 3D structure of a protein results directly from its amino acid sequence. Every protein composed of the same sequence of amino acids should have the same exact folded structure. Furthermore, we know that protein structure defines protein behavior.
Amino acid sequence → Protein structure → Protein behavior
So the question scientists have been trying to solve is, “Given an amino acid sequence, how will the protein fold?”. Solving that problem will enable us to tackle new problems like designing a protein that does what we want. Effectively, we are trying to turn this “function follows form” problem into a “form follows function” problem that we’ve been solving for ages.
CASP
In 1994, a global experiment called CASP (Critical Assessment of protein Structure Prediction) emerged to challenge the best researchers to predict how proteins fold. Every 2 years, groups compete for recognition and prestige with the ultimate goal of helping the world understand this phenomenon, which can unlock incredible potential for drug discovery.
As compute power has improved every year, better algorithms and faster iterations became possible for researchers to optimize their predictions. However, from 2006 to 2016, the accuracy of models failed to improve significantly.
In 2018, Alphabet’s DeepMind entered the competition with their expertise in deep learning. They outperformed every other model in terms of accuracy, and in 2020 they outperformed themselves again by such a significant margin that some individuals are claiming DeepMind basically solved the protein folding problem.
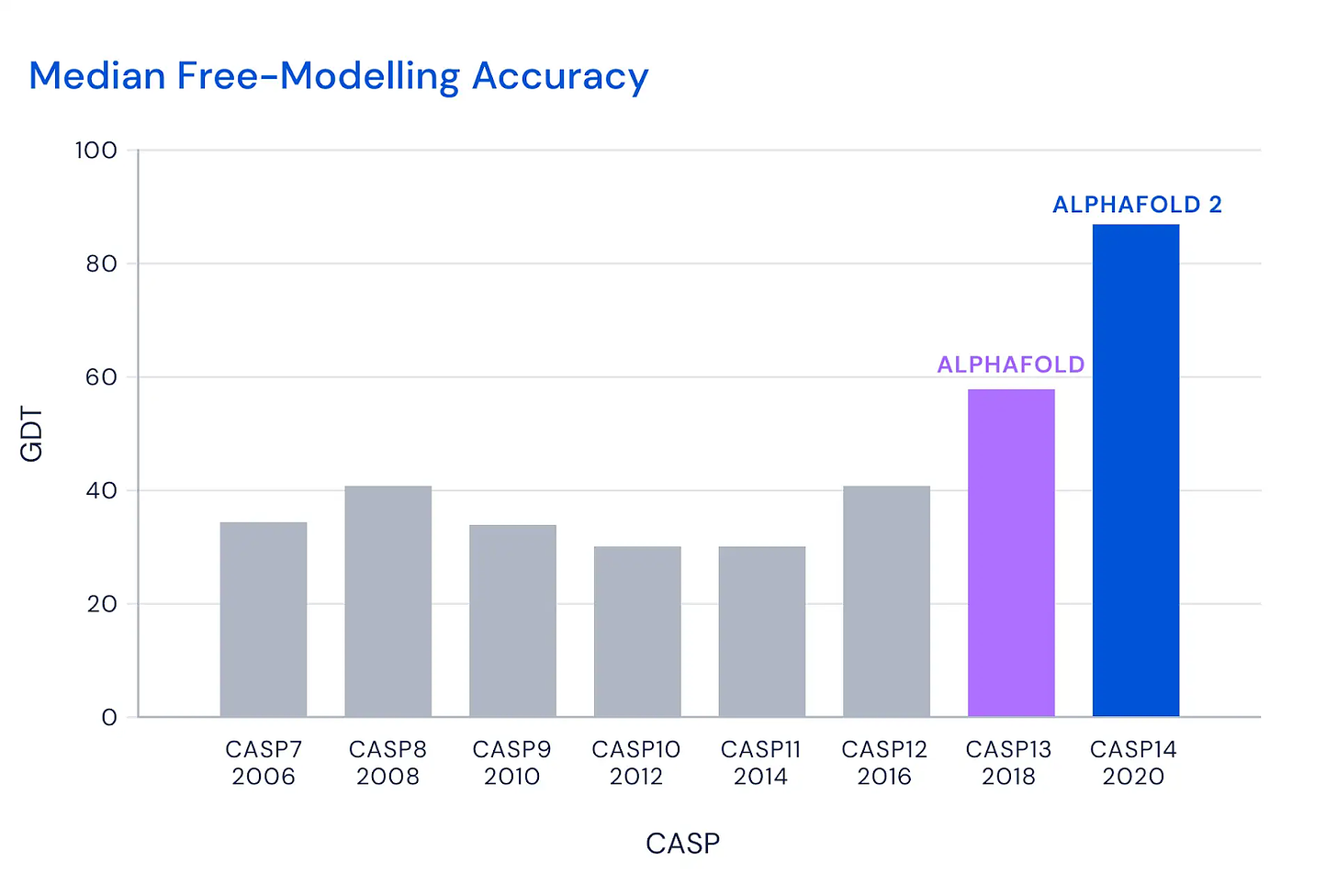
Furthermore, CASP14 had the most difficult prediction targets yet compared to any previous year. The results were so astounding that some groups like Osnat Herzberg from the University of Maryland found their own experiments to be wrong after comparing their results to those of AlphaFold 2.
Out of 146 teams competing, 1 team (DeepMind’s AlphaFold 2) outperformed every other team by a significant amount (see leftmost bar). Part of DeepMind’s success comes from their ability to use vast computing resources that others cannot access nor afford, such as 128 v3 TPU’s provided by Google’s data centers. TPU’s are Google’s custom application-specific integrated circuits (ASICs) that are used to improve and accelerate machine learning workloads. TPU’s are able to train some models 27x+ faster and 38%+ cheaper than GPU’s. DeepMind’s ability to iterate and train models faster than any other team is an advantage that clearly results in success.
Misfolding
Proteostasis is the state in which proteins are folded correctly and bodily functions are successfully completed as proteins carry materials appropriately throughout our cells.
When proteins are under stress due to various environmental reasons, they can denature, unfold, or misfold, which causes a change in behavior that can become toxic to the cell. Incorrect folding can lead to neurodegenerative diseases such as Alzheimer’s and Parkinson’s or lysosomal (abnormal build-up of toxic material) diseases such as Gaucher disease.
Heat Shock Proteins
Our body’s autoimmune system contains a clever tool to fix these problematic misfolds. The solution is Heat Shock proteins (e.g. HSP27, HSP70, etc) which are molecular chaperones that:
prevent misfolding in the first place
promote refolding of misfolded proteins
remove harmful protein aggregates
Heat Shock proteins help other proteins maintain proteostasis so our cells can function correctly.
Use Cases
Now that we understand the importance of protein folding, the next question is “What can we use these protein folding predictions for?”
Predicting protein structures can help us determine how certain mutations in amino acid sequences can cause various diseases. And, if we understand how diseases are caused, then solutions are easier to synthesize. Successful predictions can also enable designing custom proteins one day that heal abnormalities in our bodies.
Experimentally calculating a protein’s structure is expensive, time consuming, and less than perfect these days. Scientists currently use methods like X-ray Crystallography to infer the protein’s structure by shooting lasers at it and viewing its diffraction pattern. Much simpler is using DeepMind’s neural network, which can predict protein structures from amino acid sequences in just minutes with no lab setup needed.
There are ~7,000 rare diseases that we know about today. An estimated 25 million Americans (i.e. 1 in 15) have a rare disease. Orphan drugs that treat rare diseases cost around $123,000 per year per patient. Orphan drugs are expensive due to high development costs, high failure rates for orphan drugs coming to market, and small total addressable markets (high demand, low supply).
Using rough math, that means there's a ~$3T market for curing rare diseases in the U.S.
Lastly, 96% of drug development processes and 90% of clinical developments fail primarily due to a poor understanding of the target protein and a lack of experience with a specific protein family’s druggability (i.e. how well a protein binds to a drug). If improving the accuracy of protein structure prediction can move these percentages by a few points, that would have a sizable positive impact on quality of life and a significant contribution to humanity.
Conclusion
Understanding and predicting a protein’s 3D functional structure quickly and accurately will have a massive impact on our drug development processes. Protein folding is critical to our body’s functionality, and ensuring proteostasis is critical to a long and healthy human life.
We believe that improving the speed, cost, and accuracy of protein structure prediction will have positive chain-reaction effects in the pharmaceutical, medical, and genomic industries by:
reducing research and development costs
shortening research and development time
raising drug and clinical success rates
improving the risk/reward ratio for developing orphan drugs by reducing direct costs and opportunity costs
save more lives and help people live longer
Get in touch to learn more about Drawing Capital’s strategy:
References
"Peptides vs Proteins - Peptide Information - Peptide Sciences." 7 Aug. 2019, https://www.peptidesciences.com/information/peptides-vs-proteins/. Accessed 28 Dec. 2020.
"Level of structural organization of protein - Online Biology Notes." 10 Sep. 2019, https://www.onlinebiologynotes.com/level-of-structural-organization-of-protein/. Accessed 29 Dec. 2020.
"AlphaFold: a solution to a 50-year-old grand ... - DeepMind." https://deepmind.com/blog/article/alphafold-a-solution-to-a-50-year-old-grand-challenge-in-biology. Accessed 27 Dec. 2020.
"CASP14: what Google DeepMind's AlphaFold 2 really ...." 3 Dec. 2020, https://www.blopig.com/blog/2020/12/casp14-what-google-deepminds-alphafold-2-really-achieved-and-what-it-means-for-protein-folding-biology-and-bioinformatics/. Accessed 28 Dec. 2020.
"Groups Analysis: zscores - CASP14." https://predictioncenter.org/casp14/zscores_final.cgi. Accessed 28 Dec. 2020.
"Cloud TPU | Google Cloud." https://cloud.google.com/tpu. Accessed 28 Dec. 2020.
"Orphazyme | Explore Heat Shock Proteins." https://www.exploreheatshockproteins.com/. Accessed 28 Dec. 2020.
"Drug Prices for Rare Diseases Skyrocket While Big Pharma ...." 10 Sep. 2019, https://www.ahip.org/drug-prices-for-rare-diseases-skyrocket-while-big-pharma-makes-record-profits/. Accessed 28 Dec. 2020.
"Improving the odds of drug development ...." https://www.nature.com/articles/s41598-019-54849-w. Accessed 28 Dec. 2020.
This letter may not be reproduced in whole or in part without the express consent of Drawing Capital Group, LLC (“Drawing Capital”).
This letter is not an offer to sell securities of any investment fund or a solicitation of offers to buy any such securities. An investment in any strategy, including the strategy described herein, involves a high degree of risk. Past performance of these strategies is not necessarily indicative of future results. There is the possibility of loss and all investment involves risk including the loss of principal.
The information in this letter was prepared by Drawing Capital and is believed by the Drawing Capital to be reliable and has been obtained from sources believed to be reliable. Drawing Capital makes no representation as to the accuracy or completeness of such information. Opinions, estimates and projections in this letter constitute the current judgment of Drawing Capital and are subject to change without notice.
Any projections, forecasts and estimates contained in this document are necessarily speculative in nature and are based upon certain assumptions. In addition, matters they describe are subject to known (and unknown) risks, uncertainties and other unpredictable factors, many of which are beyond Drawing Capital’s control. No representations or warranties are made as to the accuracy of such forward-looking statements. It can be expected that some or all of such forward-looking assumptions will not materialize or will vary significantly from actual results. Drawing Capital has no obligation to update, modify or amend this letter or to otherwise notify a reader thereof in the event that any matter stated herein, or any opinion, projection, forecast or estimate set forth herein, changes or subsequently becomes inaccurate.